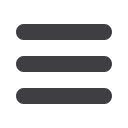
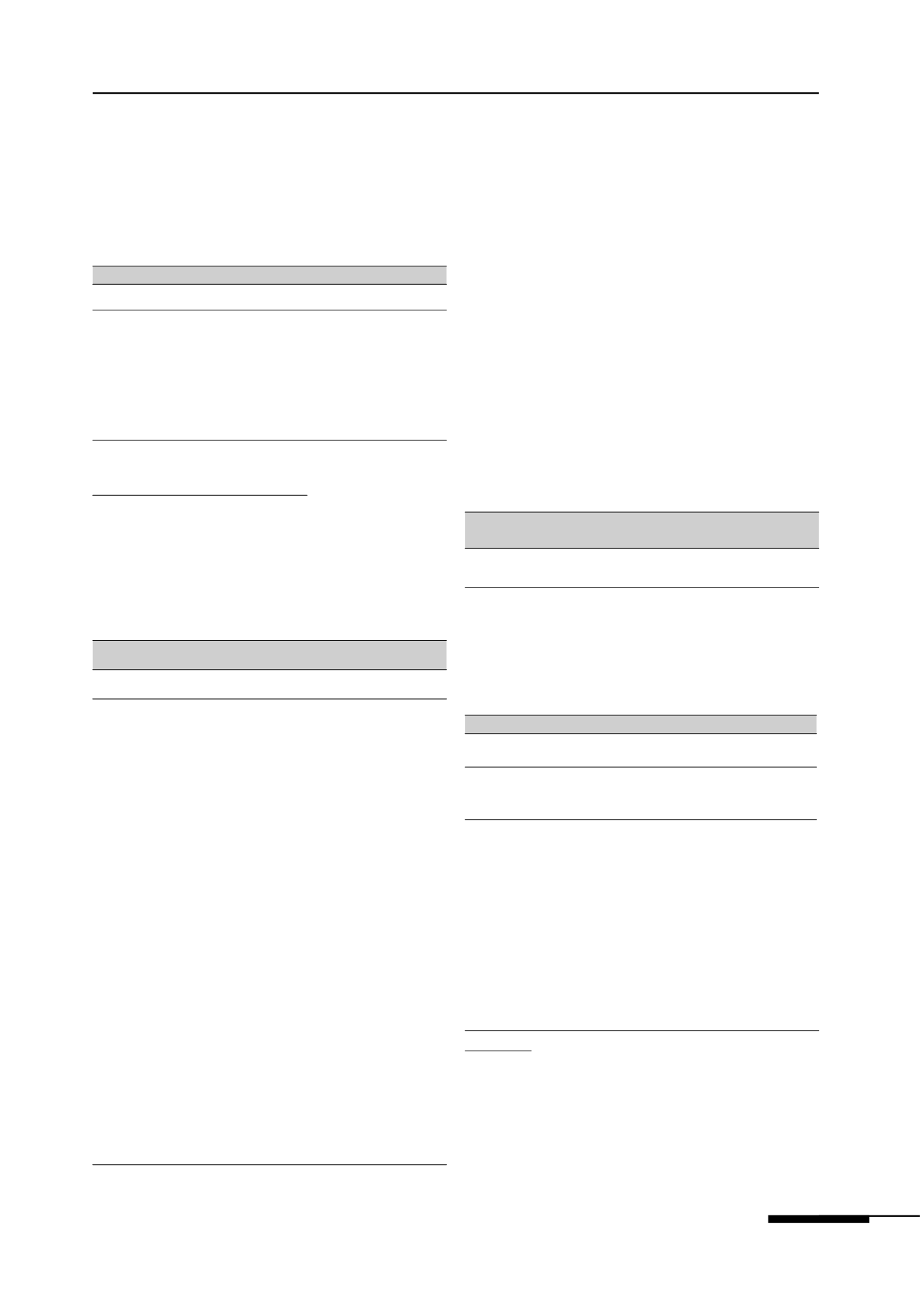
МОНГОЛЫН ХҮН АМЫН СЭТГҮҮЛ Дугаар (367) 20, 2011
83
conducted by Population Teaching and
Research Center, at School of Economic
Studies, National University of Mongolia.
The data set was collected through individual
interviews of each household.
The summary statistics of the variables are
shown in Table 2.
Table 2:
Summary Statistics
Variable
Mean Std. Dev.
Min Max
totper
4.6
2.0
1
15
age
46.0
14.5
17
90
childnum
2.0
1.5
0
10
totrev
3037.8
2036.1 130.8 1618.6
food
38.1
16.2 7.99 95.28
mig_percent
9.4
23.3
0
100
work_percent
33.4
23.2
0
100
Estimation
Ordered Logit Model (OLM)
First, I estimated the regression taking the all
chosen variable as a explanatory factor (Model
1) then if some of the regression coefficient are
been insignificant, excluding that factor from
the model and re-estimated the model (Model
2). The results are shown in Table 3.
Table 3:
OLM Estimation Result. Dependent variable:
Type of dwelling
Independent
Variables
Model 1
Model 2
totper
-0.0783 (0.040)
-0.0823
(0.040)
mig_percent
-0.0272 (0.003)
-0.0273
(0.003)
age
0.0201 (0.005)
0.0191
(0.004)
sex
-0.3417 (0.140)
-0.3352
(0.139)
educ1
0.3361 (0.407)
-
educ2
0.7443 (0.380)
0.4709
(0.204)
educ3
1.0540 (0.391)
0.7902
(0.223)
educ4
2.3010 (0.397)
2.0454
(0.230)
work
0.0138 (0.152)
-
work_percent
0.0022 (0.003)
-
childnum
-0.2624 (0.056)
-0.2599
(0.055)
totrev
0.0003 (0.000)
0.0003
(0.000)
food
-0.0087 (0.005)
-0.0088
(0.005)
LR chi-square
599.93
598.36
Prob > Chi-square
0.0000
0.0000
Pseudo R-square
0.2045
0.2040
Observations
1407
1407
Note:
() standard error. Insignificant variables are shown as bold.
Before excluding the three variables (
educ1,
work, work_percent
) the LR test was
employed. Table 4 illustrates those three
variables have no effects on the type of
dwelling. Education level being primary and
non educated have same effects on choosing
type of the conventional dwelling. The
percentage of working persons in household
has no effect on categorical dependent variable
as well. Perhaps, it is due to its skewed
distribution, the 83 per cent of total household
is non migrant; only 2 per cent of total
household is migrant. Using this kind of data,
it would be difficult to catch the significant
effect of this variable. My expectation about
the work variable was reasonably high but
the result was not good enough. Maybe, this
variable is not suitable with the chosen model
which is requiring further study.
Table 4:
Likelihood-ratio test
(Assumption: tested in
LRTEST_0)
LR chi-square (3)=1.57
Prob > chi-square = 0.6661
The Model 2 has pretty good results, all Z
and LR chi square statistics are statistically
significant. But it is important to check the
parallel regression assumption before using
this model.
Table 5:
Parallel regression assumption test results
Test type
Chi-
square
p>chi-square
df
Approximate
likelihood-ratio test
50.36
0.000
10
Brant test
56.28
0.000
10
From the Table 5, both tests show that the
parallel regression assumption can be rejected
at the 0.01 level. In this case, as a noted
by Long and Jeremy when the assumption
is rejected, alternative models should be
considered that do not impose the constraint of
parallel regression.
Multinominal Logistic Model Estimation
(MNLM)
Since we cannot use the ordinal logit
model, let’s concentrate on the MNLM. The
result is shown in Table 6.